Sensor data reproducibility is used to quantify the data quality achieved by low-cost sensors. Air quality monitoring systems used for pollutant measurement across urban and industrial areas entail numerous benefits. They provide policymakers and air quality researchers with sound solutions to fill knowledge gaps. These gaps refer to the lack of reliable data from regulatory monitors as well as satellite data.
Consensus is an important parameter for the communication of information from scientific findings. This establishes a sense of reliability for the policymakers along with the general public. In a broader sense, it is the acceptance of a hypothesis (after multiple studies) by experts in a field of study. It helps to determine whether an experiment conducted under varied conditions can lead to similar conclusions. This is a precursor to eradicating errors that stem from factors such as personal bias.
Consensus for sensor data reproducibility is achieved by close examination of the device. For instance, on exposure to a target gas with zero concentration, the sensor should provide the same reading with multiple measurements. These measurements recorded in the laboratory, as well as the site of the final installation, ensure robust calibration along with higher accuracy.
Initially, air sensors can over or underestimate pollutant concentrations as compared to a reference level monitor. This is a result of the sensitivity of these sensors toward changes in ambient air characteristics such as temperature and relative humidity. However, the calibration process along with the correction parameters refine those discrepancies leading to increased data accuracy.
Introduction to parameters for assessing sensor data
Precision, accuracy, repeatability, and reproducibility are primary aspects of determining the reliability of data. Hereby, we try to establish these concepts in the air quality monitoring system context.
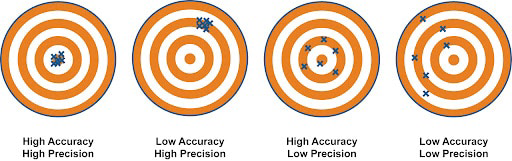
Precision and accuracy concept for multiple measurements
Precision examines the drifts between subsequent readings of the sensor. The closeness between multiple sensor readings under identical conditions determines the measurement precision. Precision tests do not take the true value to be obtained into account.
Accuracy is the closeness of the measured value to the actual value. For instance, when exposed to a 4000 ppb SO2 gas, an output of 4002 ppb is more accurate than 4300 ppb.
Reproducibility of data is often used interchangeably with repeatability. However, there are fine differences in their definitions. The factors which ideally should not affect the sensor measurements such as the location or the instrument should be the same when calculating the repeatability coefficient. However, in reproducible measurements, results should remain consistent with varying external conditions.
Repeatability is the closeness of two independent test results. These readings should be recorded under identical conditions. Scientific literature suggests the use of Normalized root mean square (NRMV) value for quantifying repeatability.
What is Reproducibility
Reproducibility is a parameter for testing measurements. It determines the consistency with which a sensor can replicate multiple measurements under different conditions. The use of multiple identical devices provides reliable insights into the extent of reproducibility. The accuracy of sensors can be further quantified by determining the standard deviation of multiple tests. It was embodied as a method of interlaboratory consistency of measurement by the American Society of Testing and Materials (ASTM). ISO 5725 further highlights the applicability of these methods for enhanced data reliability.
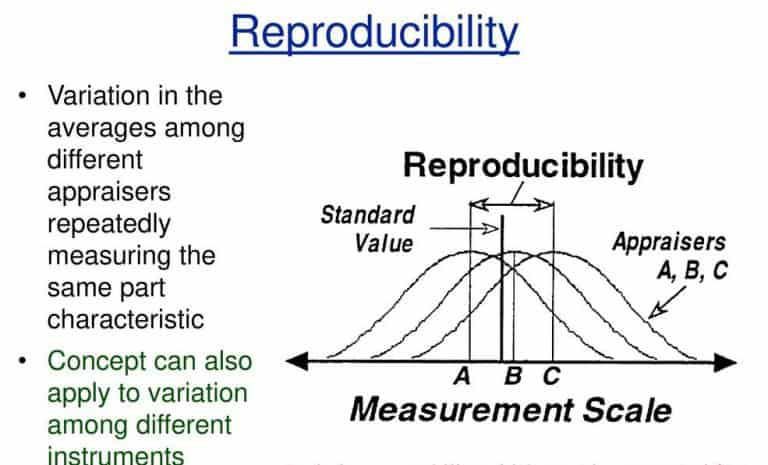
Reproducibility in scientific experiments
It is a measure of further verification of data for establishing credibility. This is largely important as far as large-scale deployment of air quality sensors is concerned. While measuring reproducibility, upon altering several factors during multiple tests, the sensor output should remain consistent. For instance, the ambient conditions such as temperature and relative humidity. For identical known gas concentrations (in accordance with NIST guidelines), the sensors should provide the same values when conditions are altered. The colocation studies on-site provide an additional base for remote data correction by adjusting according to the ambient conditions.
How does low-cost sensor data reproducibility affect reliability?
Sensor data reproducibility tests when carefully planned provide a basis for data refinement. This leads to higher reliability and data accuracy. A carefully planned integrated network of low-cost sensors and reference monitors can provide reliable results. The compact nature of low-cost devices coupled with robust calibration enables high-grade ambient pollutant monitoring.
A major benefit of measuring reproducibility is to determine the discrepancies in the sensor response and laboratory methods. Systematic errors occur due to an underlying procedural or environmental cause. These are subject to detection and quantification with the help of multiple laboratory tests. These errors can be identified by using reproducibility parameters (such as reproducibility standard deviation). Thereafter, bias and drifts can be corrected for enhanced efficiency.
State-of-the-art laboratory on reproducibility research for various pollutant sensors
The book ‘On Being a Scientist’ by the National Academy of Sciences, is essentially a guide for responsible conduct in scientific research. It outlines the responsibility of handling data and deriving actionable insights.
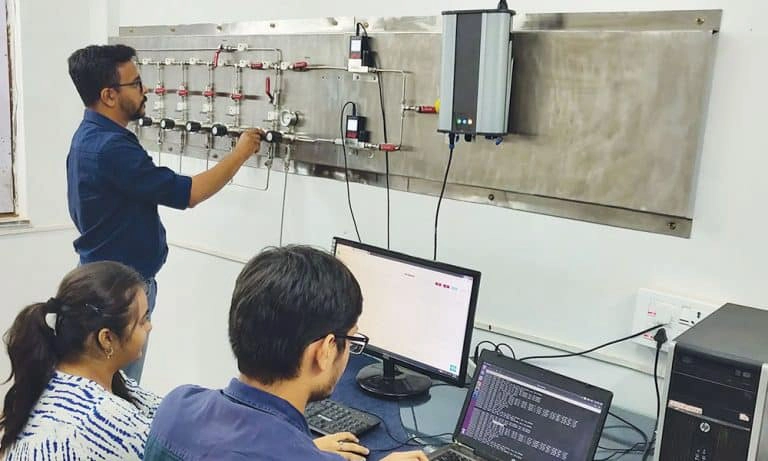
Laboratory studies for reproducibility
OIZOM’s calibration and laboratory measurement methodologies follow a similar path of inculcating the diverse aspects of accuracy determination through different methodologies. Several researchers employ the concept of correlation to determine how the sensor outputs change with altered scenarios. A study in Chile attempted to identify the correlation between reference monitor data and low-cost sensor data for PM2.5 and PM10. 1-h and 24-h average values upon correlation with the relative humidity levels in proximity provide insights into data reliability. These are helpful indicators of whether the sensor performance enhances or deteriorates with changes in humidity levels. Thereafter, the relationship between sensor discrepancy and external environmental conditions indicates whether environmental or personal bias leads to data drifts.
Sensor data reproducibility tests are crucial to eradicate internal discrepancies in the equipment. This provides a base for the multifold benefits of the laboratory calibration procedure. These include enhanced sensor accuracy and identification of underlying conditions that hinder or facilitate the reliability of the low-cost sensor monitoring system.
OIZOM Case study
OIZOM conducts case studies in order to determine the interference of diverse factors on sensor data. These studies are conducted in both laboratory and on the field, that is colocation calibration. In a fully automated system, OIZOM’s dedicated terminal provides the required features to eradicate regional interferences.
The sensor data reproducibility tests are capable of determining the sensor deviations and their underlying causes. In a laboratory environment, NIST gas cylinders are used to expose the device to a known gas. These readings recorded at 10-second intervals for 20-30 minutes provide numerous insights into the reliability aspect. This is primarily used to determine the extent of sensor drift with changes in input gas concentrations. A preliminary analysis reported a general trend of overestimation of sulfur dioxide concentration. This raw data before calibration of the sensor also reported a considerable correlation with the reference gas concentrations.
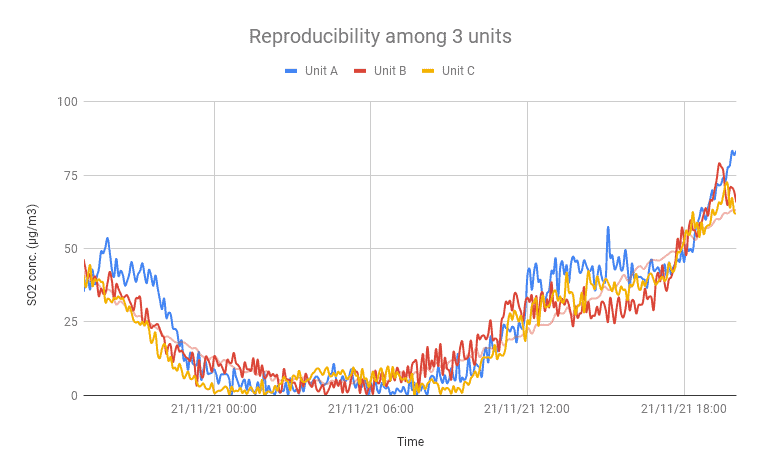
Reproducibility among multiple sensor outputs for identical input gas concentration
Low-cost sensors prove to be a crucial link for integrated air quality assessment. Supported by scientific studies and correction methodologies, the accuracy is subject to enhancement.